October 7, 2022
Figure 1. QSP improves confidence in both the compound and the target.
Reporting status of immunogenicity data components (reported vs. not reported)
Learn more about our Quantitative Systems Pharmacology Consulting Services
Predictive insights leveraging the largest, global QSP modeling team.
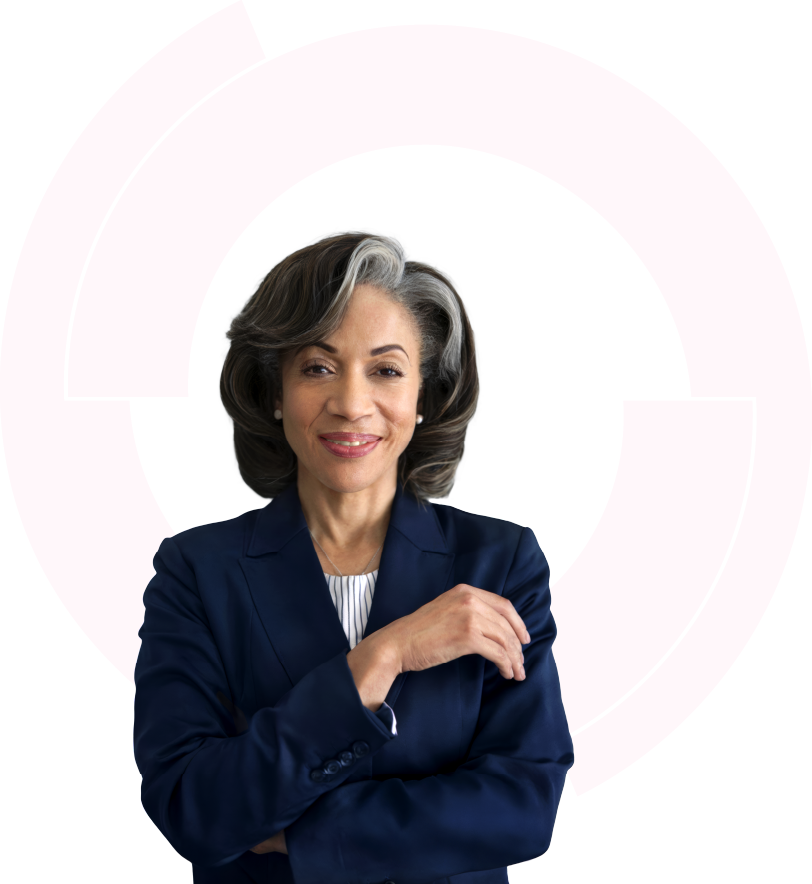
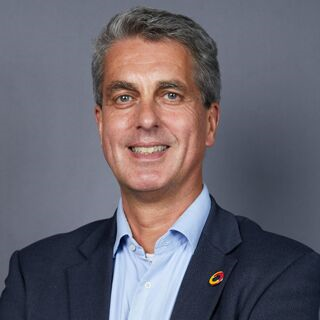
Senior Vice President and Head of Quantitative Systems Pharmacology
Piet van der Graaf is Senior Vice President and Head of Quantitative Systems Pharmacology at Certara and Professor of Systems Pharmacology at Leiden University. From 2013-2016 he was the Director of Research of the Leiden Academic Centre for Drug Research. From 1999-2013 he held various leadership positions at Pfizer in Discovery Biology, Pharmacokinetics and Drug Metabolism and Clinical Pharmacology.
He was the founding Editor-in-Chief of CPT: Pharmacometrics & Systems Pharmacology from 2012-2018 before becoming Editor-in-Chief of Clinical Pharmacology & Therapeutics. Piet received his doctorate training in clinical medicine with Nobel prize laureate Sir James Black at King’s College London. He has been awarded the 2024 Gary Neil Prize for Innovation in Drug Development from the American Society of Clinical Pharmacology and Therapeutics (ASCPT) and was the recipient of the 2021 Leadership Award from the International Society of Pharmacometrics (ISoP). Piet is an elected Fellow of the British Pharmacological Society and has published >200 articles in the area of quantitative pharmacology and drug development.
Contact Certara