March 4, 2025
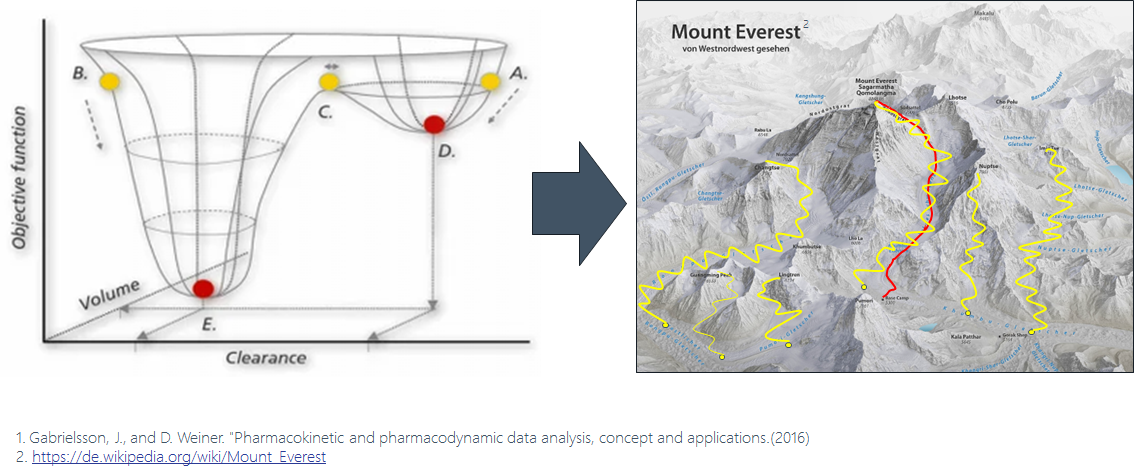
Figure 1. Identifying the best model is like climbing a mountain. There are many possible routes, but which one is the best?
Challenge
- up to 20 parameters to estimate
- difficult to identify the global minimum
Solution
- scan search space of hypotheses
- find final model representing the data set
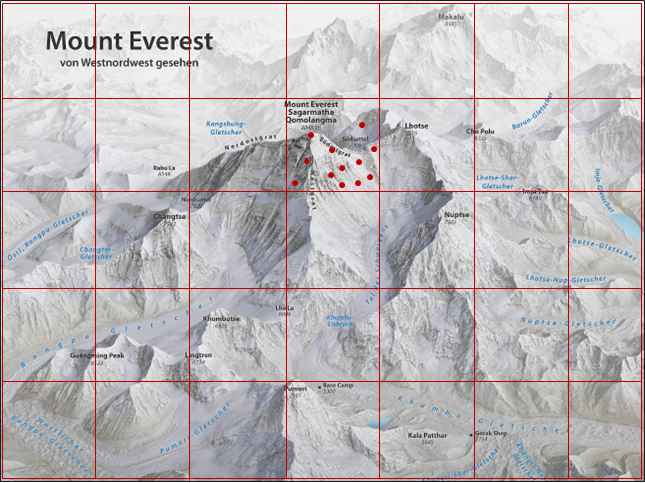
Figure 2. ML algorithms can use a non-sequential approach to model building and automatically identify the best models.
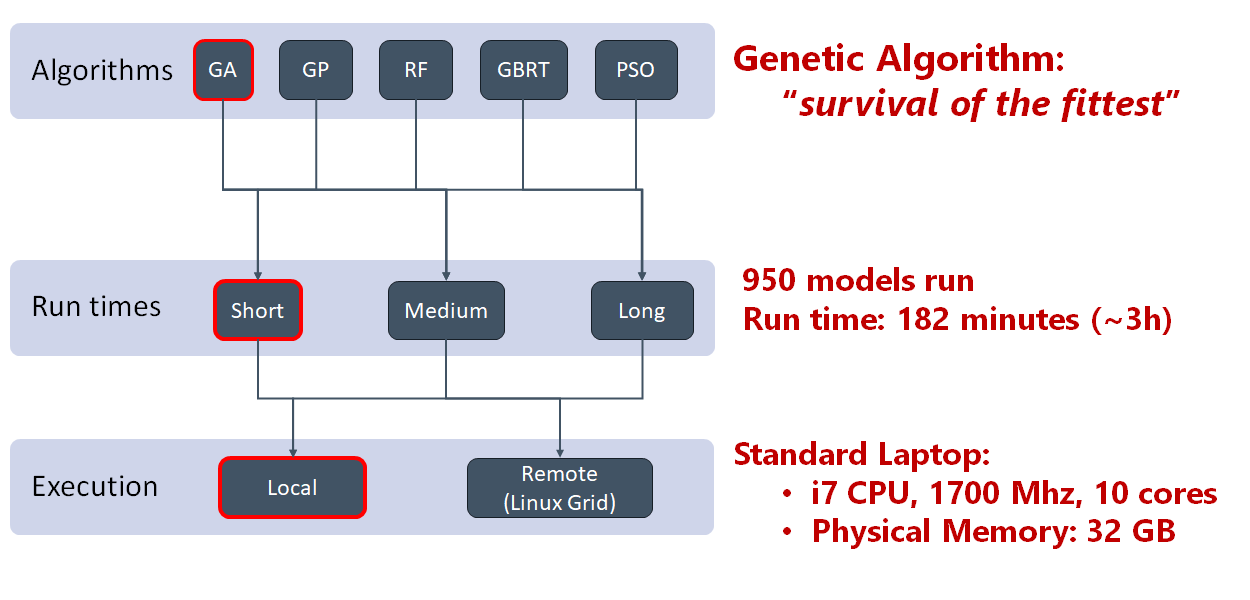
Figure 3. Machine learning algorithms can evaluate a larger number of potential models, faster than a human can.
- Stepwise model building may overlook critical interactions between model features
- ML navigated in complex parameter space
- All hypothesizes considered
- Superior model identified
- Model reviewed and accepted
- The Analyst has a key role in:
- generating hypothesis
- reviewing final model
- validating its biological plausibility
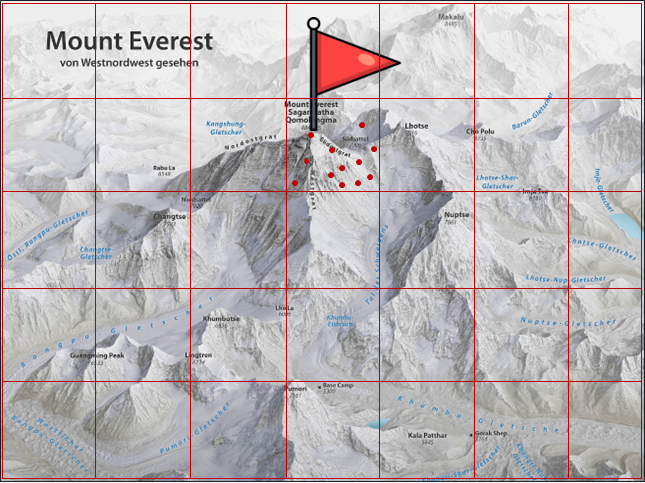
Figure 4. ML can help identify superior PK/PD models, but the role of the scientist is indispensable.
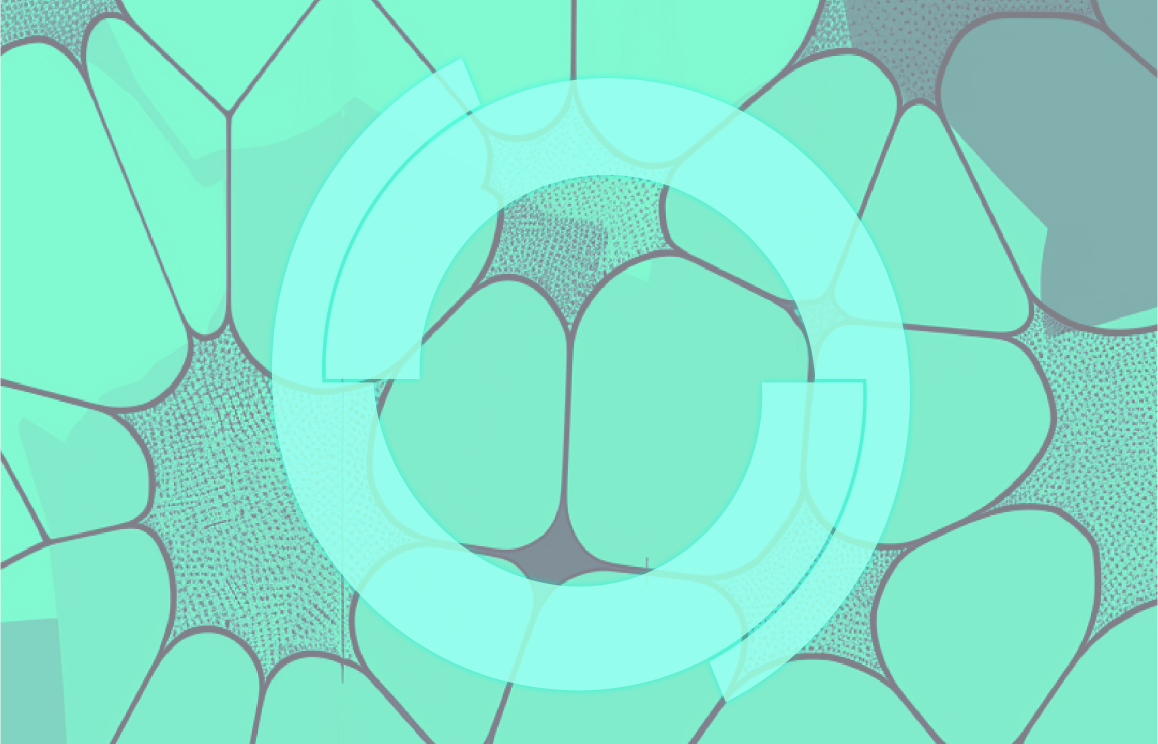
Machine Learning for Population PK Model Selection: Theory and Practice
To learn more about machine learning for pharmacometric model selection, watch this webinar.
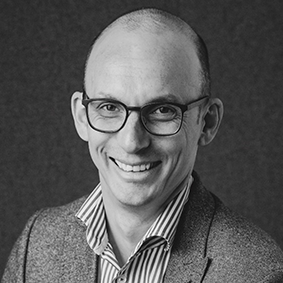
Senior Marketing Manager
Sebastian Kuchenmeister has been a Senior Marketing Manager at Certara since 2022. He is a creative marketing professional with extensive expertise in multiple marketing disciplines, campaign management, media planning and a passion for content creation and go-to market strategies. Mr. Kuchenmeister earned a Bachelor of Arts degree in Political Science from the Humboldt University in Berlin, Germany.
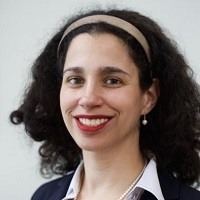
Director of Content Strategy
Dr. Suzanne Minton is the Director of Content Strategy where she leads a team of writers that develop the whip smart, educational, and persuasive content is the foundation of Certara’s thought leadership programs. She has a decade of experience in corporate marketing and has conducted biomedical research in infectious disease, cancer, pharmacology, and neurobiology. Suzanne earned a BS in biology from Duke University and a doctorate in pharmacology from the University of North Carolina at Chapel Hill.
Book a demo